
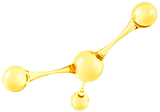
Causal AI
At Allos, we harness the power of causal relations to uncover how changes in a clinical variable can directly affect another. Causal reasoning doesn't just skim the surface with correlations: it dives deep, unraveling the threads that connect causes to their effects in a way that's both clear and actionable.

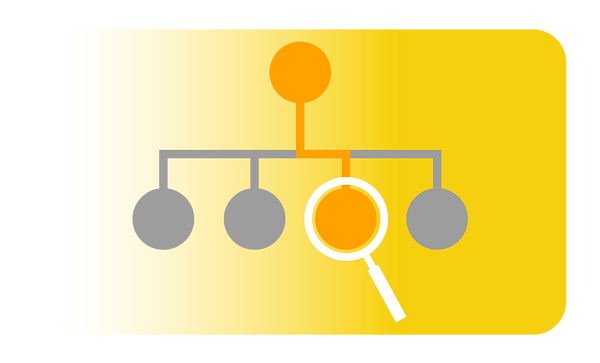
Compared to traditional AI methods, causality thrives on precision and insight. It eliminates the need for vast raw datasets, a common bottleneck in pharma, it does not require extensive training and it can accurately respond to "What if" scenario to predict future outcomes in interventional studies.
Our Causal AI model is a fusion of advanced technologies, integrating Large Language Models (LLMs) with state-of-the-art causal discovery algorithms. The insights gained are structured as causal graphs, offering a clear roadmap of how variables interact within clinical studies, enhancing our ability to develop effective and safe complex generics.
